B12 Lunch-n-learn: Learning Personal Style from Few Examples
A few weeks ago, we hosted a B12 lunch-n-learn research talk by grad student David Chuan-En Lin and professor Nik Martelaro from CMU on a question near and dear to B12’s heart: how might machines elicit style preferences from users?
Prior to the presentation, B12er Pao Siangliulue ran a reading group where we discussed David and Nik’s paper on the topic entitled Learning Personal Style from Few Examples, which they published and presented at the Designing Interactive Systems Conference 2021. They were kind enough to join B12 to present to our team, and were excited for us to record and share their talk, which you can watch here.
The problem this paper addresses is that of asking someone what visual content (e.g., images, websites) they prefer, and showing them more content that they will prefer. This problem is fundamental to the creative process: a designer’s first conversation with a new customer centers on determining what the customer’s preferences are, and it’s often hard to get past a generic “something clean, modern, and professional.” While techniques such as mood boards help facilitate a more grounded discussion, being able to quickly show a customer more of what they like to confirm a shared understanding is critical, and that’s where David and Nik’s work comes in.
The key contribution of the research is a framework called PseudoClient that, given 5–10 examples of images a user likes, can learn the user’s preferences with a higher accuracy than several baseline models. The PseudoClient model borrows from few shot learning techniques to reduce the number of examples the user has to provide, and relies on comparison and juxtaposition to visually explore both positive and negative examples.
We won’t do the paper more justice than the talk or website will, so watch and read those to learn more. The question-and-answer session was a delight, and we dove deep into future work questions such as:
-
How might we apply this approach beyond imagery to elicit style preferences on a website? This makes the problem simultaneously more complex but also provides structure on which to build additional features.
-
How might machines mediate a discussion of why a user likes something, rather than just what that user likes? Designers and researchers often contextually ask follow-up questions to understand the motivation behind a user’s preferences, and having a grammar or model to facilitate these follow-up questions would allow for a deeper understanding.
If you’re interested in answering questions like these at scale, reach out: we’d love to work with you!
Read next
See all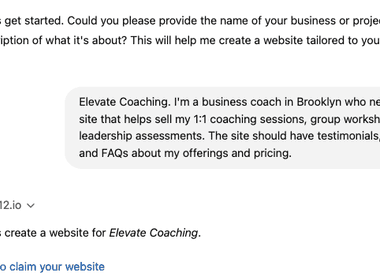
Trusted by 1M+ users: Our most personalized Website Generator yet
Build custom websites faster, add new pages in seconds, and manage multiple sites effortlessly
Read now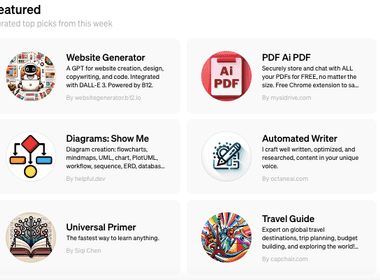
OpenAI features B12’s Website Generator in the GPT Store
Create and customize your website directly in ChatGPT using DALL-E
Read now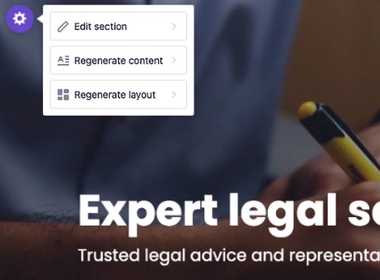
Lightning-fast website creation with B12's generative AI
Generate your tailored website in one click. To make edits, regenerate any section instantly.
Read now